Antimicrobial resistance . Drug tolerance . Bacterial heterogeneity . Treatment failure . Non-tuberculous mycobacteria
Pulmonary Infection Biology
Molecular causes of treatment failures in chronic respiratory infections
In pulmonary medicine, we face increasing numbers of chronic respiratory infections causing significant morbidity and mortality. One of them is M. abscessus, which is an emerging, extremely difficult to treat pathogen. Despite having drugs with in vitro activity against M. abscessus, multi-drug therapy often fails. Conventional drug susceptibility testing does not explain most of these treatment failures.
Our lab aims to identify bacterial mechanisms (other than drug resistance) causing treatment failure, reveal their underlying molecular biology, and provide treatment targets for new antimicrobial strategies. We combine clinical studies with bacterial genomic/proteomic profiling and novel, in our lab established, phenotyping techniques.
Large-scale assessment of drug tolerance via single-cell imaging
Drug resistance assays measure whether a bacterium can grow during antibiotic treatment, but they do not evaluate bacterial killing. Drug tolerant bacteria, which are bacteria killed at a slower rate, are very likely responsible for many treatment failures. So far, killing assessments have been cumbersome, time-intensive and are therefore not scalable. Due to these technical restrictions, our understanding of bacterial killing is poor. To overcome the current limitations, we have established an entirely new live-cell imaging pipeline, allowing us to study bacterial killing in hundreds of different experimental conditions at once (figure 1). We can now apply this pipeline to study molecular mechanisms underlying bacterial killing, identify sterilising drug targets, and define the relevance of drug tolerance. This platform could also help in bacterial characterisation and clinical decision making in the future.
Systematic assessment of drug-drug interactions in mycobacteria
Due to rapidly emerging de novo drug resistance in mycobacteria, single-drug treatments are not useful. Multi-drug regimens are mainly based on individual drug effects rather than their properties in combination. However, synergistic drug combinations (the combination is more effective than the sum of individual drugs) could be strategically used, whereas antagonistic combinations (the combination is less effective than the sum of individual drugs) may restrict bacterial clearance. We systematically assess thousands of drug-drug interactions by growth and killing measurements, thereby characterising general principles of drug synergisms and antagonisms in mycobacteria.
Genome-proteome-phenotype inference in Mycobacterium abscessus
We have previously applied a genome-wide association study approach, which uses the diversity of clinical isolates to identify molecular mechanisms underlying phenotypic variability (GWAS, figure 2). However, this approach does not account for epistasis or non-genetic (non-heritable) mechanisms. Via proteomic profiling of several hundred M. abscessus isolates, we aim to generate multi-dimensional models capturing protein function (genomics) and quantity (proteomics) to improve phenotype prediction, and thus our mechanistic understanding driving natural bacterial variation in patients.
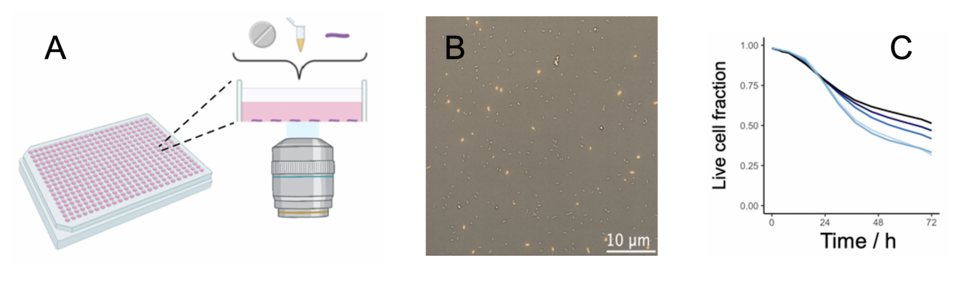
Figure 1: Bacterial live-cell imaging platform (A) Live bacteria are immobilised at the well bottom of 384-well plates and exposed to different conditions. (B) Each field is imaged every 3h over 3 days (yellow: dead cells). About 5 Mio. bacteria are automatically segmented per plate and can be tracked throughout the experiment. (C) Time-kill curve of M. abscessus treated with different amikacin concentrations.
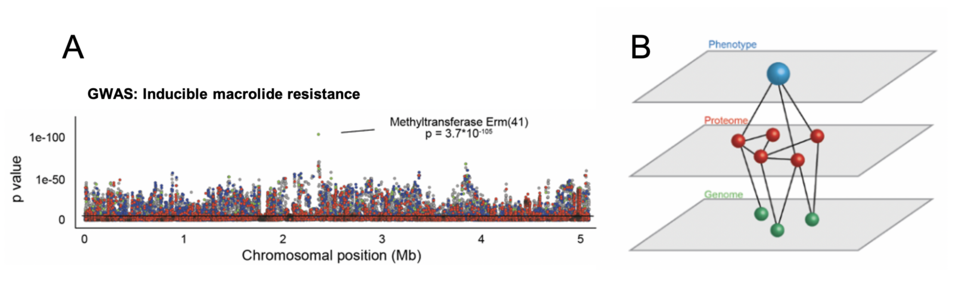
Figure 2: Inferring phenotypic variability from whole genome sequencing and proteomics (A) Genome-wide association study identifies erm(41) as a mechanism for inducible macrolide resistance (B) Quantitative proteomic data of ~350 isolates are used to generate functional (epistatic) networks. Combinations of genomic and proteomic signatures are then applied to predict mycobacterial phenotypes.